CompSust Open Graduate Seminar (COGS)
The COGS will focus on disseminating work of graduate students in the computational sustainability network. The format will be short (~30 minute) presentations with plenty of time for open discussion. All are welcome to attend. The series is sponsored by CompSustNet, with support from the National Science Foundation's Expeditions in Computing program.
For information about previous semesters, please see the past COGS list.
See also the Computational Sustainability Virtual Seminar Series.
COGS Schedule
Seminar Details
Ming Jin, UC Berkeley
Fri Feb 7, 2020, 1:30–2:30pm EST (UTC-5)
Title: Robust Data Analytics for Safety-Critical Systems
Abstract: In the future, data and algorithms will play an increasingly important role in solving societal-scale problems, from improving people's living conditions to modernizing the electric grid. Unlike traditional machine learning tasks such as image classification, these problems involve complex physical systems that are safety-critical with humans included, where data could be scarce, corrupted, and even adversarial. Consequently, these systems require robustness guarantees against the uncertainty that arises from (1) anomalous inputs or adversarial attacks, and (2) human interventions; moreover, the algorithms need to be scalable and data-efficient. In this talk I will focus on my recent works in designing computational methods that meet these requirements. The central theme is to develop robust algorithms with formal performance guarantees for complex systems, with emphasis on urban infrastructures that prioritize human values.
Bio: Ming Jin is a postdoctoral researcher in the Department of Industrial Engineering and Operations Research at UC Berkeley. He received his doctoral degree from the EECS department at UC Berkeley in 2017. His current research interests include optimization, learning and control for safety-critical systems.
Amrita Gupta, Georgia Tech
Fri Feb 21, 2020, 1:30–2:30pm EST (UTC-5)
Title: Budget-Constrained Demand-Weighted Network Design for Resilient Infrastructure
Abstract: Our work is motivated by an important network design problem in climate adaptation. As floods become more frequent and severe due to climate change, it is increasingly crucial that road infrastructure be strategically upgraded to support post-disaster recovery efforts and normal functionality. We focus on the problem of allocating a fixed budget towards restoring edges to maximize the satisfied travel demand between locations in a network, which we formalize as the budget constrained prize-collecting Steiner forest problem. We prove that the satisfiable travel demand objective exhibits restricted supermodularity over forests, and utilize this property to design an iterative algorithm based on maximizing successive modular lower bounds for the objective that finds better solutions than a baseline greedy approach. We also propose an extremely fast heuristic for maximizing modular functions subject to knapsack and graph matroid constraints that can be used as a subroutine in the iterative algorithm, or as a standalone method that matches the greedy baseline in terms of quality but is orders of magnitude faster. We evaluate the algorithms on synthetic data, and apply them to a real-world instance of retrofitting the Senegal national road network against flooding.
Bio: Amrita is a 5th year PhD student in the School of Computational Science and Engineering at the Georgia Institute of Technology. She works with Professor Bistra Dilkina, developing models, algorithms and tools for resource allocation problems in the emerging field of Computational Sustainability.
Bryan Wilder, Harvard
Fri Mar 6, 2020, 1:30–2:30pm EST (UTC-5)
Title: Group-Fairness in Influence Maximization
Abstract: Influence maximization is a widely used model for information dissemination in social networks. Recent work has employed such interventions across a wide range of social problems, spanning public health, substance abuse, and international development (to name a few examples). A critical but understudied question is whether the benefits of such interventions are fairly distributed across different groups in the population; e.g., avoiding discrimination with respect to sensitive attributes such as race or gender. Drawing on legal and game-theoretic concepts, we introduce formal definitions of fairness in influence maximization. We provide an algorithmic framework to find solutions which satisfy fairness constraints, and in the process improve the state of the art for general multi-objective submodular maximization problems. Experimental results on real data from an HIV prevention intervention for homeless youth show that standard influence maximization techniques oftentimes neglect smaller groups which contribute less to overall utility, resulting in a disparity which our proposed algorithms substantially reduce.
Bio: Bryan Wilder is a fifth-year PhD student in Computer Science at Harvard University, where he is advised by Milind Tambe. His research focuses on the intersection of optimization and machine learning, with the goal of improving interventions that serve vulnerable populations. His work has received or been nominated for best paper awards at ICML and AAMAS, and was a finalist for the INFORMS Doing Good with Good OR competition. He is supported by a NSF Graduate Research Fellowship.
Tegan Maharaj, Mila - Quebec AI Institute
Fri Mar 20, 2020, 1:30–2:30pm EDT (UTC-4)
Title: Thoughts and Experiments at the Intersection of Theoretical Ecology and Deep Learning
Abstract: The current research is at the intersection of theoretical ecology and deep learning. I'll give an intro to theoretical ecology/ecosystem modelling, and then I'll talk about ways these two fields can benefit from each other by giving some examples of work that I'm doing or plan to do. I'll zoom in particular on some early results in using meta-learning to learn representations of chaotic dynamical systems.
Bio: Tegan is affiliated with Mila. Tegan is broadly interested in understanding the effects on learning behavior and generalization of varying "what goes into" deep models. In her research so far this has included multimodal input data, regularization, and different forms of feedback or environment, with applications to video, natural language, and climate data. She is concerned and passionate about AI ethics, safety, and the application of ML to environmental management, health, and social welfare.
Sara Beery, CalTech
Fri Apr 3, 2020, 1:30–2:30pm EDT (UTC-4)
Title: Improving Computer Vision for Camera Traps: Leveraging Practitioner Insight to Build Solutions for Real-World Challenges
Abstract: Camera traps are widely used to monitor animal populations and behavior, and generate vast amounts of data. There is a demonstrated need for machine learning models that can automate the process of detecting and classifying animals in camera trap images. Previous work has shown exciting results on automated species classification in camera trap data, but further analysis has shown that these results do not generalize to new cameras or new geographical regions, and struggle to categorize rare species or poor quality images. Consequently, very few organizations have successfully deployed machine learning tools for camera trap image review. I will discuss my recent work tackling these real-world challenges with improved model architectures, data de-siloing, and data augmentation methods, and building accessible tools for biologists with Microsoft AI for Earth and Wildlife Insights.
Bio: Sara Beery is a PhD Candidate and NSF Fellow at Caltech, advised by Pietro Persona. Her research focuses on machine learning and computer vision for biodiversity monitoring, particularly for detection and recognition of animal species in challenging camera trap data at a global scale. She works closely with Microsoft AI for Earth and Google Research/Wildlife Insights where she helps turn her research into usable tools for the biodiversity community.
Riccardo Pressiani, UIC
Fri Apr 17, 2020, 1:30–2:30pm EDT (UTC-4)
Title: A Comprehensive Hardware and Software Solution for Identifying Wild Animals' Behaviors
Abstract: Activity recognition and, more generally, behavior inference tasks are gaining a lot of interest. Much of it is work in the context of human behavior. New available tracking technologies for wild animals are generating datasets that indirectly may provide information about animal behavior. In this work, we propose a method for classifying these data into behavioral annotations, particularly collective behaviors of a social group. Our method is based on sequence analysis with a direct encoding of the interactions of a group of wild animals.
This method was the foundation of a larger research work that we conducted titled "On how to track social individuals behaviors via a mixed HW/SW solution".
In this work, we propose a new comprehensive solution composed of a sensor system to remotely extract data from social wild animals and an improved version of the supervised machine learning framework to classify animal behaviors.
We evaluate our approach on real-world datasets, including a new one that we gathered during a field trip in Kenya in August 2018, showing significant accuracy improvements over existing sensor technologies and baseline methods.
Bio: Riccardo is Co-Founder and Software Engineer at Wepo, a software consulting company specialized in Artificial Intelligence and Machine Learning. He completed a double Master's Degree program in Computer Science and Engineering with Politecnico di Milano (Italy) and the University of Illinois at Chicago.
Riccardo's research thesis was about developing a comprehensive system (HW & SW) to identify wild animals' behaviors through sensors and machine learning.
His main interests are software engineering, cloud-based applications, and the IoT world, with a focus on how to apply data science techniques to sensors data.
Sujith Mangalathu, UCLA
Fri May 1, 2020, 1:30–2:30pm EDT (UTC-4)
Title: Analytics-Driven Models to Support the Design and Service-Life Management of Infrastructure Systems
Abstract: The rapid increase in computational power, coupled with the improvements in numerical modeling and availability of performance data provides a unique opportunity to enhance the resilience and sustainability of spatially distributed infrastructure systems. This talk introduces the development of analytics-driven models to support the design and service-life management of infrastructure systems. Machine learning methods are used to perform rapid post-hazard-event assessments of the damage and functional state of impacted infrastructure systems and components. The analytics-driven framework is presented as a viable alternative to the traditional approach, which relies heavily on visual inspections and subjective estimates of the state of an infrastructure system. The machine learning methods presented in this talk are used to (1) develop bridge-specific fragility curves for more than 24,000 concrete box-girder California bridges, and (2) generate real-time estimates of the spatial distribution and severity of building damage following an earthquake.
Bio: Sujith Mangalathu is a research scientist in Equifax in the field of adaptive machine learning and graph analysis for risk assessment. He obtained his Ph.D. from Georgia Institute of Technology, in 2017. He also spent two years as a post-doctoral research scholar at University of California, Los Angeles. His research is focused on the application of machine learning techniques for the risk assessment of structural and infrastructure systems with a special emphasis on bridge systems. He is also working on the performance-based grouping of structures for regional risk assessment and statistical decisions that support post-earthquake emergency response. He strives to develop innovative methods for regional risk assessment of structural systems using advanced statistical and machine learning techniques.
Samuel Buteau, Dalhousie University
Fri May 15, 2020, 1:30–2:30pm EDT (UTC-4)
Title: Applying Machine Learning to Understand Lithium-ion Cells
Abstract: Lithium-ion cells have a huge role to play in electrifying transportation and transitioning the grid to use solar and wind energy. To be competitive, these cells need to pack a lot of energy at low cost and have lifetimes of many years. However, sustaining good performance for many years means that the development cycle is way too long unless lifetimes of decades can be estimated in months (hopefully weeks or days). Previous work has shown qualitatively that this type of prediction can be done, but the work remains of taking in lots of experimental data and producing a reliable quantitative prediction model. In this talk, I attempt to combine the generalization properties of physics-based models with the power of neural networks. I will also discuss the infrastructure required to keep track of the experimental data, the design of each cell, and model the impact of chemistry on lifetime degradation. Finally, I will showcase the open-source implementation of these ideas.
Bio: Sam is a 4th year PhD student from Dalhousie University working with Professor Jeff Dahn, pursuing the task of gathering years worth of high-quality experimental research on lithium-ion cells and unleashing machine learning techniques on it to gain some predictive power and speed up the research cycle.
COGS Program Committee
Hari Prasanna Das (UC Berkeley)
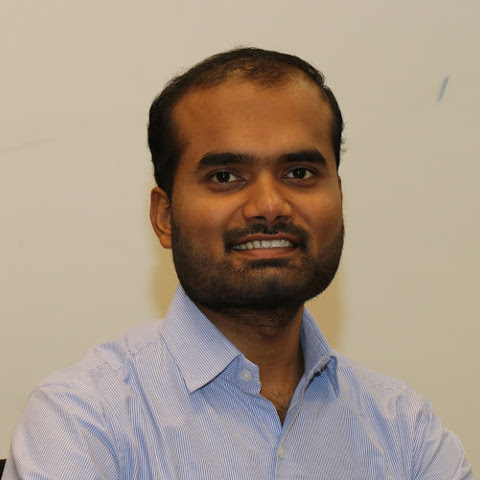
Hari is a Ph.D. student in the Department of Electrical Engineering and Computer Sciences at University of California, Berkeley, advised by Prof. Costas J. Spanos. Hari is also a Graduate Student Researcher for the Singapore-Berkeley Building Efficiency and Sustainability in the Tropics (SinBerBEST) program. His current research interests are probabilistic machine learning, including topics in generative modeling, approximate inference, deep learning and their applications relating to smart buildings and climate change.
Priya Donti (CMU)
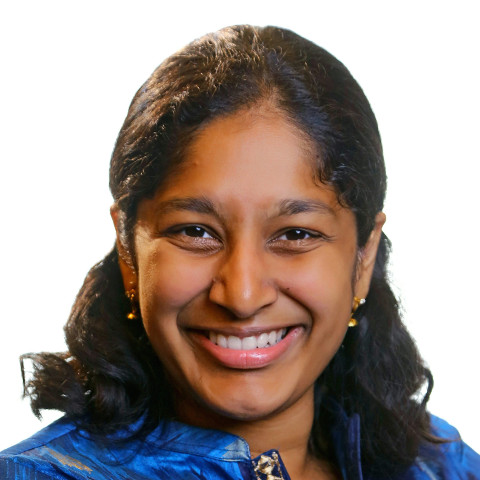
Priya is a Ph.D. student in Computer Science and Public Policy at Carnegie Mellon University (co-advised by Zico Kolter and Inês Azevedo) and a US Department of Energy Computational Science Graduate Fellow. Her work focuses on incorporating domain knowledge such as power system physics into machine learning techniques. She is also a co-chair of Climate Change AI, an initiative to facilitate meaningful work at the intersection of climate change and machine learning.
Neil Gaikwad (MIT)
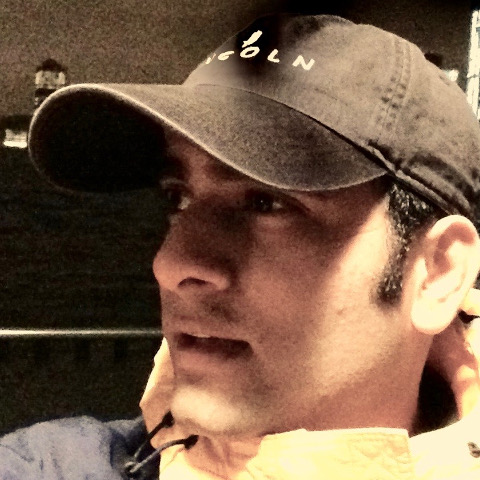
Neil Gaikwad is a doctoral scholar at the Massachusetts Institute of Technology, specializing in human-centered machine learning and social computing for sustainability. He is currently developing human-centered machine learning for decoding the impact of social and the Earth's physical processes on global food security. His honors include the Facebook Research Fellowship, the MIT Graduate Teaching Award, and the Karl Taylor Compton Prize, the highest student award presented by MIT in recognition of excellent achievements in citizenship and devotion to the welfare of MIT. For more information visit http://web.media.mit.edu/~gaikwad.
Kevin Winner (UMass)
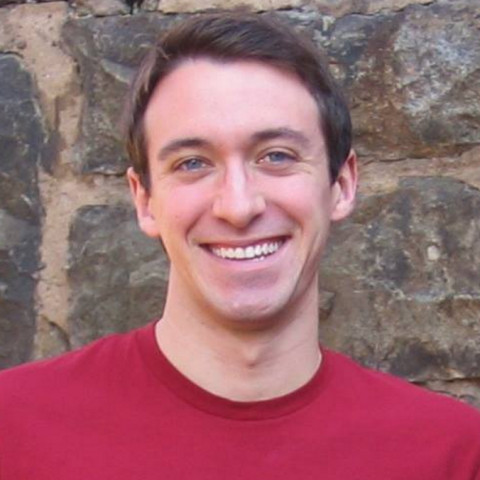
Kevin is a postdoc in the Center for Biodiversity and Global Change at Yale University, working with Dr. Walter Jetz. He is currently applying machine learning techniques to model global-scale patterns of biodiversity and species distributions. He recently completed his PhD in computer science at the University of Massachusetts, Amherst, where his research focused on novel demography models for populations of unmarked individuals.
Lily Xu (Harvard)
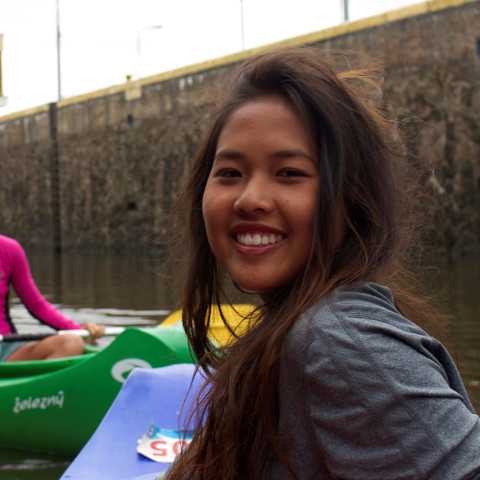
Lily Xu is a second-year PhD student at Harvard University, advised by Prof. Milind Tambe. Her research focuses on applications of artificial intelligence — specifically in online learning and game theory — to wildlife conservation. Lily graduated from Dartmouth College in 2018, where she studied computer science and Spanish.
Past committee members
- Sebastian Ament (Cornell)
- Nima Dolatnia (OSU)
- Amrita Gupta (GATech)
- Neal Jean (Stanford)
- Bryan Wilder (USC)