Past CompSust Open Graduate Seminar (COGS)
The COGS will focus on disseminating work of graduate students in the computational sustainability network. The format will be short (~30 minute) presentations with plenty of time for open discussion. All are welcome to attend. The series is sponsored by CompSustNet, with support from the National Science Foundation's Expeditions in Computing program.
For current seminar information, please see the Spring 2020 listing.
See also the Computational Sustainability Virtual Seminar Series.
2018 Spring | |||
---|---|---|---|
Date/Time | Speaker | Title | Links |
Fri Apr 20, 2018, 1:30–2:30pm EDT (UTC-4) | Yexiang Xue, Cornell University | Avicaching: a Two-stage Game for Bias Reduction in Citizen Science | |
Fri May 4, 2018, 1:30–2:30pm EDT (UTC-4) | Haifeng Xu, University of Southern California | Strategic Coordination of Human Patrollers and UAVs with Signaling for Security Games | |
Fri Jun 1, 2018, 1:30–2:30pm EDT (UTC-4) | Kevin Winner, University of Massachusetts Amherst | Inference and Learning with Generating Functions for Models of Population Dynamics | |
2018 Fall | |||
Date/Time | Speaker | Title | Links |
Fri Sep 21, 2018, 1:30–2:30pm EDT (UTC-4) | Di Chen, Cornell University | End-to-End learning for the Deep Multivariate Probit Model | |
Fri Oct 5, 2018, 1:30–2:30pm EDT (UTC-4) | Priya L. Donti, Carnegie Mellon University | Inverse Optimal Power Flow: Assessing the Vulnerability of Power Grid Data | |
Fri Oct 19, 2018, 1:30–2:30pm EDT (UTC-4) | David Abel, Brown University | Bandit Based Solar Panel Control | |
Fri Nov 2, 2018, 1:30–2:30pm EDT (UTC-4) | Andrew Perrault, University of Southern California | Experiential Preference Elicitation for Autonomous HVAC Systems | |
Fri Nov 30, 2018, 1:30–2:30pm EST (UTC-5) | Neil Newman, University of Southern California | Designing and Evolving an Electronic Agricultural Marketplace in Uganda | |
2019 Spring | |||
Date/Time | Speaker | Title | Links |
Fri Jan 25, 2019, 1:30–2:30pm EST (UTC-5) | Lynn Kaack, Carnegie Mellon University | Truck traffic monitoring with satellite images | |
Fri Feb 6, 2019, 1:30–2:30pm EST (UTC-5) | Roel Dobbe, New York University | An Integrative Approach to Data-Driven Control in Electric Distribution Networks | |
Fri Mar 8, 2019, 1:30–2:30pm EST (UTC-5) | Sherrie Wang, Stanford University | Weakly Supervised Learning for Satellite Imagery: Applications in Crop Mapping | |
Fri Mar 22, 2019, 1:30–2:30pm EDT (UTC-4) | Paulo Orenstein, Stanford University | Improving Subseasonal Forecasting in the Western U.S. with Machine Learning | |
Fri Apr 19, 2019, 1:30–2:30pm EDT (UTC-4) | Anson Kahng, Carnegie Mellon University | Virtual Democracy and Food Rescue | |
Fri Apr 26, 2019, 1:30–2:30pm EDT (UTC-4) | Amanda Coston, Carnegie Mellon University | Counterfactual Risk Assessments for Child Welfare Screening | |
Fri May 3, 2019, 1:30–2:30pm EDT (UTC-4) | Jackson Killian, University of Southern California | Learning to Prescribe Interventions for Tuberculosis Patients using Digital Adherence Data |
2018 Spring COGS Details
Yexiang Xue, Cornell University
Fri Apr 20, 2018, 1:30–2:30pm EDT (UTC-4)
Title: Avicaching: a Two-stage Game for Bias Reduction in Citizen Science
Abstract: In this talk, I will discuss how game theory can help reduce the data bias problem in citizen science. Citizen science projects have been very successful at collecting rich datasets across different domains. However, the data collected by the citizen scientists are often biased, aligned more directly with the participants' preferences rather than scientific objectives. We introduce a general methodology to improve the scientific quality of the data collected by citizen scientists. Our approach uses incentives to shift the interests of citizen scientists to be more aligned with the goal of obtaining unbiased samples from the field, thus improving the quality of the data collected. We formulate the problem using the so-called Principal-Agent framework, which requires an integration of learning, to obtain the parameters that govern the individual behavior of the citizen scientists (the agents), with reasoning, to search for an optimal incentive allocation to achieve the goal of the principal (the organizer of the citizen science program). We apply our methodology to eBird, a well-established citizen science program of the Cornell Lab of Ornithology for the collection of bird observations, as a game-based web application, called Avicaching. Our field results show that our Avicaching incentives are remarkably effective at steering the bird watchers' efforts to explore under-sampled areas and hence alleviate the data bias problem in eBird. At the end of my talk, I will briefly discuss how the Institute of Computational Sustainability enables me to develop this fruitful line of multidisciplinary research, collaborating with wonderful domain experts.
This is joint work with Ian Davies, Daniel Fink and Christopher Wood from the Cornell Lab of Ornithology and Carla P. Gomes from the Department of Computer Science, Cornell University.
Bio: Yexiang Xue is a Ph.D. candidate in the Department of Computer Science at Cornell University, working with Professors Carla Gomes and Bart Selman. Upon graduation, he will join Purdue University as an assistant professor in computer science starting Fall 2018. His research aims at combining large-scale constraint-based reasoning and optimization with state-of-the-art machine learning techniques to enable intelligent agents to make optimal decisions in high-dimensional and uncertain real-world applications. More specifically, his research focuses on scalable and accurate probabilistic reasoning techniques, statistical modeling of data, and robust decision-making under uncertainty. Mr. Xue's work is motivated by key problems across multiple scientific domains, including artificial intelligence, machine learning, renewable energy, materials science, citizen science, urban computing, and ecology, with an emphasis on developing cross-cutting computational methods for applications in the areas of computational sustainability and scientific discovery. Mr. Xue's work received the Innovative Application Award at IAAI-17 and was featured as the cover article and the Editor's Choice in the journal Combinatorial Science of the American Chemical Society.
Haifeng Xu, University of Southern California
Fri May 4, 2018, 1:30–2:30pm EDT (UTC-4)
Title: Strategic Coordination of Human Patrollers and UAVs with Signaling for Security Games
Abstract: The past decade has seen significant interest of using game theory to model the strategic interactions between defenders and attackers (a.k.a., security games). This has also led to the deployment of optimized defender strategies in several real-world applications including, e.g., patrol route design for wildlife conservation. Most of these works consider only the allocation of human patrollers. In this talk, I will discuss a different problem that concerns the optimal coordination of human patrollers and mobile sensors (e.g., UAVs) for better defense. This is partially motivated by the rapidly growing recent trend in using UAVs to combat poaching. I will explain the key differences between human patrollers and UAVs as security resources, and show how to improve defense by empowering human patrollers with the most natural two functionalities of UAVs: monitoring and strategic signaling.
Bio: Haifeng Xu is a PhD candidate in the Computer Science Department at the University of Southern California, advised by Milind Tambe and Shaddin Dughmi. His research interests include artificial intelligence, computational game theory, algorithms, and applied machine learning. He focuses on developing theoretically grounded approaches that also have real-world impact. Haifeng is a recipient of the 2017 Google PhD fellowship and the 2017 USC CAMS prize for excellence in research. His work has received the 2016 AAMAS best student paper award and the 2016 SecMas Workshop best paper award.
Kevin Winner, University of Massachusetts Amherst
Fri Jun 1, 2018, 1:30–2:30pm EDT (UTC-4)
Title: Inference and Learning with Generating Functions for Models of Population Dynamics
Abstract: Graphical models with integer-valued latent variables appear in numerous applications, but they are particularly ubiquitous when modeling the growth and decay of partially observed populations over time. However, typical inference and learning algorithms for discrete latent variable models fail when the support of the latent variables is unbounded, as it is in our scenario. Tractable approximate algorithms exist, but they require a priori assumptions about the population size and don't provide any theoretical results about the degree of the approximation, meaning it can be difficult to tune these assumptions or to detect when they fail.
By comparison, our new family of inference and learning algorithms based on generating functions are capable of solving these problems faster than existing techniques and are the first to do so exactly. The approach has two key ideas: 1) to adopt a representation of probability distributions based on probability generating functions, and 2) to use ideas from forward mode automatic differentiation (autodiff) to evaluate the generating functions. We have focused on the application of these concepts to modeling population dynamics, but the core concepts are broadly applicable to any model with unbounded integer latent variables (for idea #1) or to any function evaluation problem requiring nested derivatives (for idea #2).
In this talk, I will present the foundations of PGF-based inference and learning, including how to adapt your favorite inference/learning algorithm to use a generating function representation and how to evaluate the resultant complex recursive functions using high-order nested autodiff. I will also present some of our ongoing and planned work in this domain. The talk will run about 40 minutes, with an extended 20 minutes question and discussion segment following the talk.
We are also planning an upcoming code release, so if you'd like more information or to get an early release of the package, please contact me!
2018 Fall COGS Details
Di Chen, Cornell University
Fri Sep 21, 2018, 1:30–2:30pm EDT (UTC-4)
Title: End-to-End learning for the Deep Multivariate Probit Model
Abstract: Understanding multi-entity interactions is a central question in many real-world applications. For example, in computational sustainability, it is important to understand the spatial distribution of species and how species interact with each other and their environment, for developing conservation plans. In computer vision, the detections of multiple objects are often correlated because of the shared background and scenario. In natural language processing, a text often has several correlated labels in terms of its topic, emotion, and semantic meaning.
The multivariate probit model (MVP) is a popular classic model for studying binary responses of multiple entities. Nevertheless, the computational challenge of learning the MVP model, given that its likelihood involves integrating over a multi-dimensional constrained space of latent variables, significantly limits its application in practice.
In this talk, I will present a flexible deep generalization of the classic MVP, the Deep Multivariate Probit Model (DMVP), which is an end-to-end learning scheme that uses an efficient parallel sampling process of the multivariate probit model to exploit GPU-boosted deep neural networks. We show that when applied to multi-entity modelling problems, which are natural DMVP applications, DMVP trains faster than classical MVP, by at least an order of magnitude, captures rich correlations among entities, and further improves the joint likelihood of entities compared with several competitive models.
The talk will run about 30 minutes, with an extended 20 minutes question and discussion segment following the talk. I released my code on the bitbucket and you are welcome to use DMVP to explore the interesting multi-entity correlation in your own domain.
Bio: Di Chen is a second-year Ph.D. student in the Department of Computer Science at Cornell University, advised by Carla P. Gomes. His research includes solving structured prediction, multi-entity modeling and covariate shift using state-of-the-art deep learning techniques.
Priya L. Donti, Carnegie Mellon University
Fri Oct 5, 2018, 1:30–2:30pm EDT (UTC-4)
Title: Inverse Optimal Power Flow: Assessing the Vulnerability of Power Grid Data
Abstract: In deregulated electricity markets, gaining knowledge of critical information such as grid structure, generator bidding cost curves, and nodal power demands could pose risks to market efficiency and cybersecurity. It is thus in the best interest of grid operators to protect critical information from the general public, so as to ensure fair, efficient, and safe market operation. At the same time, system operators such as PJM and governmental agencies such as the EPA regularly publish information about public market quantities such as energy prices and generator power outputs, which could potentially expose private data.
We seek to investigate the question of whether and to what extent privately-held market information is potentially exposed by published market information, given our knowledge that private and public parameters are related via an optimization problem called AC optimal power flow (ACOPF). Specifically, we formulate an algorithm called "inverse optimal power flow" (Inverse OPF) that uses gradient descent-based methods implemented within a neural network to learn unknown market and grid parameters. The eventual goal is to quantify the potential risks of having this information exposed.
The talk will run about 30 minutes, with 30 minutes of discussion following the talk. I welcome any feedback about the research methods as they currently stand, as well as advice regarding quantification of the real-world effects of our findings.
Bio: Priya Donti is a third-year Ph.D. student in the Computer Science Department and the Department of Engineering & Public Policy at Carnegie Mellon University, co-advised by Zico Kolter and Inês Azevedo. Her research is at the intersection of deep learning and energy policy, exploring topics such as marginal emissions prediction, grid data vulnerability, and end-to-end task-based approaches for coordinating between grid forecasting and control.
David Abel, Brown University
Fri Oct 19, 2018, 1:30–2:30pm EDT (UTC-4)
Title: Bandit Based Solar Panel Control
Abstract: Solar panels sustainably harvest energy from the sun. To improve performance, panels are often equipped with a tracking mechanism that computes the sun's position in the sky throughout the day. Based on the tracker's estimate of the sun's location, a controller orients the panel to minimize the angle of incidence between solar radiant energy and the photovoltaic cells on the surface of the panel, increasing total energy harvested. Prior work has developed efficient tracking algorithms that accurately compute the sun's location to facilitate solar tracking and control. However, always pointing a panel directly at the sun does not account for diffuse irradiance in the sky, reflected irradiance from the ground and surrounding surfaces, power required to reorient the panel, shading effects from neighboring panels and foliage, or changing weather conditions (such as clouds), all of which are contributing factors to the total energy harvested by a fleet of solar panels. In this work, we show that a bandit-based approach can increase the total energy harvested by solar panels by learning to dynamically account for such other factors. Our contribution is threefold: (1) the development of a test bed based on typical solar and irradiance models for experimenting with solar panel control using a variety of learning methods, (2) simulated validation that bandit algorithms can effectively learn to control solar panels, and (3) the design and construction of an intelligent solar panel prototype that learns to angle itself using bandit algorithms. I further discuss my reflections on this project, and the experiences our group had in attempting to translate our prototype from the lab and into the hands of solar farms.
Bio: David Abel is a fourth year PhD candidate in CS at Brown University, advised by Michael Littman. His research studies the role abstraction plays in sequential decision making, with a focus on uniting the theories of computation, abstraction, and reinforcement learning. Before Brown, he received his bachelors in CS and Philosophy from Carleton College.
Andrew Perrault, University of Southern California
Fri Nov 2, 2018, 1:30–2:30pm EDT (UTC-4)
Title: Experiential Preference Elicitation for Autonomous HVAC Systems
Abstract: AI systems that act on behalf of users require knowledge of user preferences, which can be acquired by preference elicitation. In many settings, users can more easily and accurately respond to preference queries reflecting their current, or recently experienced, context (e.g., state of the environment), than those reflecting contexts further removed. We develop and study a formal model of experiential elicitation (EE) in which query costs and response noise are state-dependent. EE settings tightly couple the problems of control and elicitation. We provide some analysis of this abstract model, and illustrate its applicability in household heating/cooling management. We propose the use of relative value queries, asking the user to compare the immediate utility of two states, whose difficulty is related to the degree and recency of a user's experience with those states. We develop a Gaussian process-based approach for modeling user preferences in dynamic EE domains and show that it accrues higher reward than several natural baselines.
Bio: Andrew Perrault is a postdoctoral scholar at University of Southern California, supervised by Milind Tambe. His research focuses on decision-making and market design in environmental applications of artificial intelligence, especially in domains where gathering information is expensive. Andrew received his PhD in Computer Science from University of Toronto, advised by Craig Boutilier, and his BA from Cornell. He is the co-founder and co-lead developer at theschoolfund.org, a non-profit that crowdfunds scholarships for secondary school students in developing countries.
Neil Newman, University of Southern California
Fri Nov 30, 2018, 1:30–2:30pm EST (UTC-5)
Title: Designing and Evolving an Electronic Agricultural Marketplace in Uganda
Abstract: Motivated by inefficiencies in agricultural markets in developing countries, we designed Kudu, an electronic marketplace that facilitates agricultural trade in Uganda. Traders and farmers post what they want to buy and sell on Kudu using a feature phone, and Kudu proposes profitable matches. Users are supported by in-village services and a call center. Over the past two and a half years, more than 20,000 users have signed up for Kudu; these users have performed verified transactions with an aggregate value of over $1.9 million USD.
Bio: Neil Newman is a Computer Science PhD student supervised by Kevin Leyton-Brown at the University of British Columbia. He works on market clearing problems. Examples includes the station repacking problem in the FCC's Incentive Auction for radio spectrum, and Kudu, an electronic market for agricultural trade in developing countries.
2019 Spring COGS Details
Lynn Kaack, Carnegie Mellon University
Fri Jan 25, 2019, 1:30–2:30pm EST (UTC-5)
Title: Truck traffic monitoring with satellite images
Abstract: The road freight sector is responsible for a large and growing share of greenhouse gas emissions, but reliable data on the amount of freight that is moved on roads are scarce. Many low- and middle-income countries have limited ground- based traffic monitoring and freight surveying activities. We show that we can use an object detection network to count trucks in satellite images and predict average daily truck traffic from those counts. In this proof of concept, we describe a complete model, test the uncertainty of the estimation, and discuss the transfer to developing countries.
Bio: Lynn Kaack is a PhD Candidate in the Department of Engineering and Public Policy at Carnegie Mellon University (CMU). She holds a Master's in Machine Learning from CMU and a Bachelor and Master of Science in Physics from Free University of Berlin, Germany. Her research focuses on policy analysis and data science of problems related to energy and climate change mitigation.
Roel Dobbe, New York University
Fri Feb 6, 2019, 1:30–2:30pm EST (UTC-5)
Title: An Integrative Approach to Data-Driven Control in Electric Distribution Networks
Abstract: Ubiquitous computing, algorithms, better devices, connectivity, and the ability to collect, store and probe large amounts of data are all becoming new commodities -- commodities that are the key ingredients to enabling new forms of automation and innovation in critical infrastructures. The question is then: how can we integrate data-driven techniques to improve and extend the capabilities of existing critical infrastructure, while safeguarding important values such as safety or privacy? In this talk, this question forms the driver for modernizing electric distribution networks to deal with higher levels of renewable generation and electrification.
We integrate concepts from control theory, machine learning, optimization, information theory and differential privacy to make concrete contributions in this context:
(1) decentralizing optimal power flow, by reconstructing the solution to centralized problems with locally available information, (2) providing a formal framework to assess learning-based decentralized policies and determine optimal communication strategies, by adopting a rate distortion approach, and (3) enabling the protection of sensitive information in the objectives and constraints of distributed optimization and control problems, by integrating a differential privacy mechanism.
We conclude with an overview on the inherent accumulation of bias and error in data-driven decision-making, and a call for assessing one's epistemology and engaging with domain experts and beneficiaries to steer the design of systems towards promoting safe, beneficial and just outcomes.
Bio: Roel's research addresses the development, analysis, integration and governance of data-driven systems. His PhD work combined optimization, machine learning and control theory to enable monitoring and control of safety-critical systems, including energy & power systems and cancer diagnosis and treatment. His diverse background led him to examine the ways in which values and stakeholder perspectives are represented in the process of designing and deploying AI and algorithmic decision-making and control systems. Roel is passionate about developing practices to help engineers and computer scientists engage more closely both with impacted communities and scholars in the social sciences, and to better contend with serious questions of ethics and governance. Towards this end, Roel founded Graduates for Engaged and Extended Scholarship around Computing & Engineering (GEESE); a student organization stimulating graduate students across all disciplines studying or developing technologies to take a broader lens at their field of study and engage across disciplines.
Sherrie Wang, Stanford University
Fri Mar 8, 2019, 1:30–2:30pm EST (UTC-5)
Title: Weakly Supervised Learning for Satellite Imagery: Applications in Crop Mapping
Abstract: Feeding 7 billion people — a number likely to surpass 9 billion by 2050 — will require smarter agriculture. Knowing where crops grow worldwide is a crucial first step. Today, this information is acquired through on-the-ground surveys, which take a long time, require many people, and are tough to conduct in the countries where data is needed most. Tomorrow, it will be possible to harness satellite imagery and machine learning to decrease the cost and difficulty of mapping this information at scale. However, one main challenge in applying the tools of machine learning to crop type mapping is the low quantity of ground truth labels on which to train state-of-the-art methods (e.g. deep learning). This talk will offer a window into how unsupervised and weakly supervised learning methods can help us bridge this label gap and understand which crops are grown where.
Bio: Sherrie is a 4th year PhD student at Stanford's Institute for Computational and Mathematical Engineering (ICME), advised by Professor David Lobell at the Center on Food Security and the Environment. Her research focuses on developing semi-supervised and unsupervised methods for remote sensing data to enable understanding of food systems and their interaction with the environment at a large scale.
Paulo Orenstein, Stanford University
Fri Mar 22, 2019, 1:30–2:30pm EDT (UTC-4)
Title: Improving Subseasonal Forecasting in the Western U.S. with Machine Learning
Abstract: To improve the accuracy of long-term forecasts, the Bureau of Reclamation and the National Oceanic and Atmospheric Administration launched the Subseasonal Climate Forecast Rodeo, a year-long real-time forecasting challenge, in which participants aimed to skillfully predict temperature and precipitation in the western U.S. two to four weeks and four to six weeks in advance. Here we present and evaluate our machine learning approach to the Rodeo. Our system is an ensemble of two regression models, and exceeds that of the top Rodeo competitor as well as the government baselines for each target variable and forecast horizon.
Bio: Paulo Orenstein is a PhD Candidate in the Department of Statistics at Stanford University. He holds a Master's in Mathematics and a Bachelor of Science in Economics, both form PUC-Rio, in Brazil. His research focuses on the interplay between statistics, probability, and computation, particularly as they apply to high-dimensional Bayesian models and Monte Carlo methods.
Anson Kahng, Carnegie Mellon University
Fri Apr 19, 2019, 1:30–2:30pm EDT (UTC-4)
Title: Virtual Democracy and Food Rescue
Abstract: Virtual democracy is an approach to automating decisions, by learning models of the preferences of individual people, and, at runtime, aggregating the predicted preferences of those people on the dilemma at hand. One of the key questions is which aggregation method — or voting rule — to use; we offer a novel statistical viewpoint that provides guidance. Specifically, we seek voting rules that are robust to prediction errors, in that their output on people's true preferences is likely to coincide with their output on noisy estimates thereof. We prove that the classic Borda count rule is robust in this sense, whereas any voting rule belonging to the wide family of pairwise-majority consistent rules is not. Our empirical results further support, and more precisely measure, the robustness of Borda count. Lastly, we consider the application of virtual democracy to the domain of food rescue, or matching food donations to needy recipients.
Bio: Anson is a third-year PhD student in the Computer Science Department at Carnegie Mellon University, where he is advised by Ariel Procaccia. He works on theoretical problems at the intersection of computer science and economics, particularly in computational social choice. Recently, he has worked on voting aggregation for noisy votes, impartial voting mechanisms, virtual democracy, and liquid democracy.
Amanda Coston, Carnegie Mellon University
Fri Apr 26, 2019, 1:30–2:30pm EDT (UTC-4)
Title: Counterfactual Risk Assessments for Child Welfare Screening
Abstract: Risk assessments and other algorithmic decision-making systems are increasingly used in high-stakes applications such as criminal justice, consumer lending, and child welfare screening decisions. Many risk assessment models are trained on observational data where historical interventions may have affected the observed outcomes. Our research investigates how these observational risk models may be biased in the case of child welfare screening decisions, and we propose counterfactual risk assessments that account for the intervention affects.
Bio: Amanda is a joint PhD student in Machine Learning and Public Policy at Carnegie Mellon University. She is broadly interested in how machine learning can solve problems of societal interest, and her research areas include algorithmic fairness, causal inference, and machine learning for healthcare.
Jackson Killian, University of Southern California
Fri May 3, 2019, 1:30–2:30pm EDT (UTC-4)
Title: Learning to Prescribe Interventions for Tuberculosis Patients using Digital Adherence Data
Abstract: Digital Adherence Technologies (DATs) are an increasingly popular method for verifying patient adherence to many medications. We analyze data from one city served by 99DOTS, a phone-call-based DAT deployed for Tuberculosis (TB) treatment in India where nearly 3 million people are afflicted with the disease each year. The data contains nearly 17,000 patients and 2.1M phone calls. We lay the groundwork for learning from this real-world data, including a method for avoiding the effects of unobserved interventions in training data used for machine learning. We then construct a deep learning model, demonstrate its interpretability, and show how it can be adapted and trained in three different clinical scenarios to better target and improve patient care. In the real-time risk prediction setting our model could be used to proactively intervene with 21% more patients and before 76% more missed doses than current heuristic baselines. For outcome prediction, our model performs 40% better than baseline methods, allowing cities to target more resources to clinics with a heavier burden of patients at risk of failure. Finally, we present a case study demonstrating how our model can be trained in an end-to-end decision focused learning setting to achieve 15% better solution quality in an example decision problem faced by health workers.
Bio: Jackson Killian is a PhD student in computer science at the University of Southern California, where he is co-advised by Milind Tambe and Bistra Dilkina. His research focuses on explainable machine learning in healthcare, motivated by applications to interventions for traditionally underserved or difficult to treat populations. He is supported by a NSF Graduate Research Fellowship.
COGS Program Committee
Hari Prasanna Das (UC Berkeley)
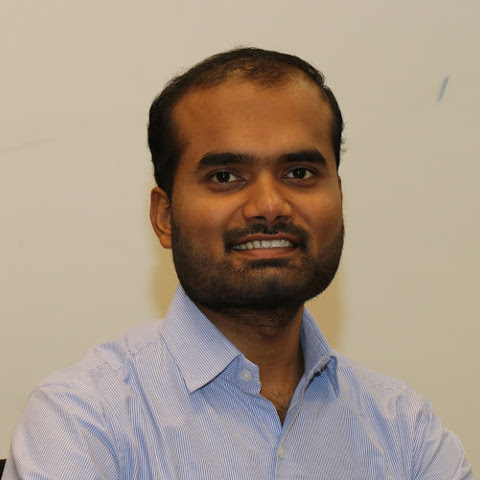
Hari is a Ph.D. student in the Department of Electrical Engineering and Computer Sciences at University of California, Berkeley, advised by Prof. Costas J. Spanos. Hari is also a Graduate Student Researcher for the Singapore-Berkeley Building Efficiency and Sustainability in the Tropics (SinBerBEST) program. His current research interests are probabilistic machine learning, including topics in generative modeling, approximate inference, deep learning and their applications relating to smart buildings and climate change.
Priya Donti (CMU)
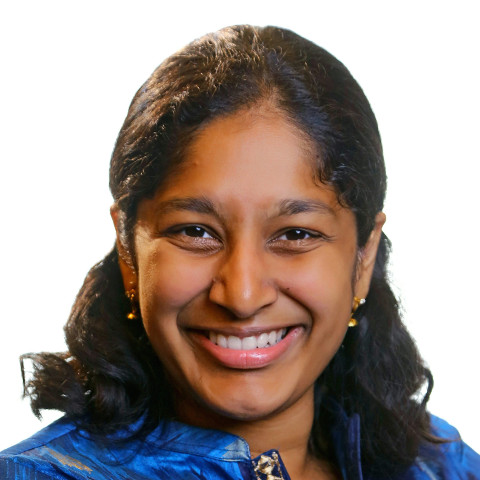
Priya is a Ph.D. student in Computer Science and Public Policy at Carnegie Mellon University (co-advised by Zico Kolter and Inês Azevedo) and a US Department of Energy Computational Science Graduate Fellow. Her work focuses on incorporating domain knowledge such as power system physics into machine learning techniques. She is also a co-chair of Climate Change AI, an initiative to facilitate meaningful work at the intersection of climate change and machine learning.
Neil Gaikwad (MIT)
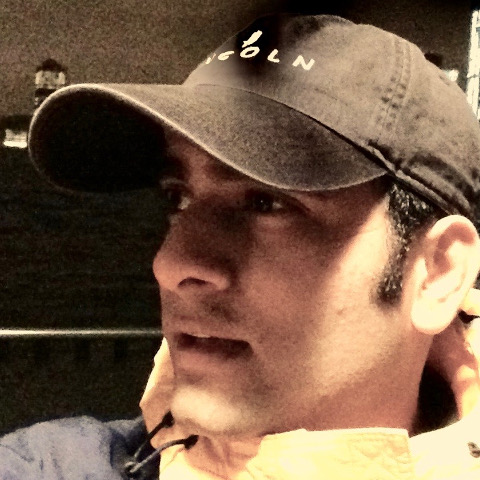
Neil Gaikwad is a doctoral scholar at the Massachusetts Institute of Technology, specializing in human-centered machine learning and social computing for sustainability. He is currently developing human-centered machine learning for decoding the impact of social and the Earth's physical processes on global food security. His honors include the Facebook Research Fellowship, the MIT Graduate Teaching Award, and the Karl Taylor Compton Prize, the highest student award presented by MIT in recognition of excellent achievements in citizenship and devotion to the welfare of MIT. For more information visit http://web.media.mit.edu/~gaikwad.
Kevin Winner (UMass)
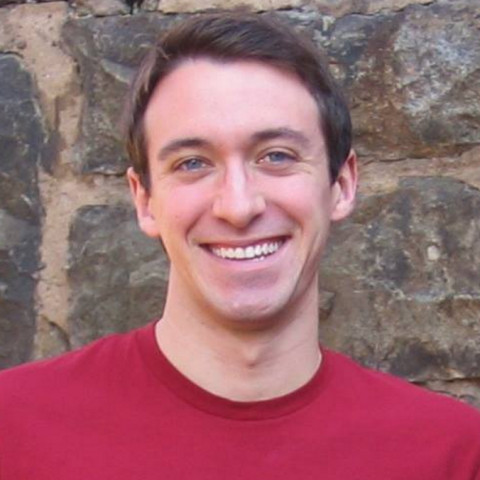
Kevin is a postdoc in the Center for Biodiversity and Global Change at Yale University, working with Dr. Walter Jetz. He is currently applying machine learning techniques to model global-scale patterns of biodiversity and species distributions. He recently completed his PhD in computer science at the University of Massachusetts, Amherst, where his research focused on novel demography models for populations of unmarked individuals.
Lily Xu (Harvard)
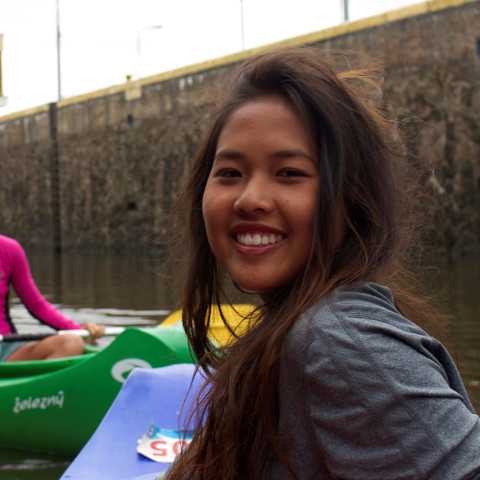
Lily Xu is a second-year PhD student at Harvard University, advised by Prof. Milind Tambe. Her research focuses on applications of artificial intelligence — specifically in online learning and game theory — to wildlife conservation. Lily graduated from Dartmouth College in 2018, where she studied computer science and Spanish.
Past committee members
- Sebastian Ament (Cornell)
- Nima Dolatnia (OSU)
- Amrita Gupta (GATech)
- Neal Jean (Stanford)
- Bryan Wilder (USC)