Conference Program
CompSust DC 2022 will take place online.
Friday, March 11, 2022
EST | CST | PST | |
---|---|---|---|
11:00am – 12:05pm | 10:00am – 11:05am | 8:00am – 9:05am | Opening Talk Opening Keynote Presentation: Dr. Volodymyr Kuleshov (Cornell Tech) |
12:05pm – 12:15pm | 11:05am – 11:15am | 9:05am – 9:15am | Break |
12:15pm – 1:15pm | 11:15am – 12:15pm | 9:15am – 10:15am | |
1:15pm – 2:00pm | 12:15pm – 1:00pm | 10:15am – 11:00am | Lunch Break |
2:00pm – 2:30pm | 1:00pm – 1:30pm | 11:00am – 11:30am | Invited Talk: Ritwik Gupta (University of California, Berkeley) |
2:30pm – 3:30pm | 1:30pm – 2:30pm | 11:30am – 12:30pm | Keynote Presentation: Dr. Claire Monteleoni (University of Colorado, Boulder) |
3:30pm – 3:45pm | 2:30pm – 2:45pm | 12:30pm – 12:45pm | Break |
3:45pm – 4:45pm | 2:45pm – 3:45pm | 12:45pm – 1:45pm |
Saturday, March 12, 2022
EST | CST | PST | |
---|---|---|---|
11:00am – 11:45am | 10:00am – 10:45am | 8:00am – 8:45am | |
11:45am – 12:00pm | 10:45am – 11:00am | 8:45am – 9:00am | Break |
12:00pm – 1:15pm | 11:00am – 12:15pm | 9:00am – 10:15am | Networking on gather.town |
1:15pm – 2:00pm | 12:15pm – 1:00pm | 10:15am – 11:00am | Lunch Break |
2:00pm – 3:00pm | 1:00pm – 2:00pm | 11:00am – 12:00pm | Keynote Presentation: Dr. Arun Majumdar (Stanford University) |
3:00pm – 3:15pm | 2:00pm – 2:15pm | 12:00pm – 12:15pm | Break |
3:15pm – 4:00pm | 2:15pm – 3:00pm | 12:15pm – 1:00pm | |
4:00pm – 5:00pm | 3:00pm – 4:00pm | 1:00pm – 2:00pm | Closing Keynote: Dr. Victor Anton (Wildlife.ai) |
5:00pm | 4:00pm | 2:00pm | Closing Remarks |
Keynote speakers
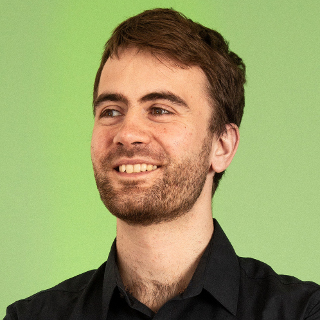
Dr. Volodymyr Kuleshov (Cornell Tech)
Towards a sustainable food supply chain powered by artificial intelligence
Abstract: About 30–40% of food produced worldwide is wasted. This represents a $165B loss to the US economy and poses major environmental problems: for example, it is estimated that food waste contributes to up to 25% of all greenhouse gas emissions. This talk explores how artificial intelligence can be used to automate decisions across the food supply chain in order to reduce waste and increase access to nutritious and affordable food. We describe how supply chain automation can be cast as a model-based control problem and present novel planning algorithms that benefit from a principled treatment of model uncertainty. We also describe how these algorithms can be deployed within an intelligent decision support system that assists supermarket operators in performing perishable inventory management. This system is currently deployed across hundreds supermarkets in the US (handling ~2% of US produce volume) and has led to waste reductions of up to 50%. We hope that this talk will bring the food waste problem to the attention of the machine learning community.
Bio: Volodymyr Kuleshov is an Assistant Professor at the Jacobs Technion-Cornell Institute at Cornell Tech and in the Computer Science Department at Cornell University. He obtained his bachelor's in Mathematics and Computer Science from McGill University, and his Ph.D. in Computer Science from Stanford University, where he was the recipient of the Arthur Samuel Best Thesis Award.
Kuleshov's research interests are in the field of machine learning and its applications in scientific discovery, health, and sustainability. His work has been featured in Nature Biotechnology, Nature Medicine, Nature Communications and Scientific American, and was awarded an NSERC Post-Graduate Fellowship and a Stanford Graduate Fellowship. He is the co-founder and Chief Technologist at Afresh, a startup focused on automating the food supply chain using AI in order to reduce food waste.
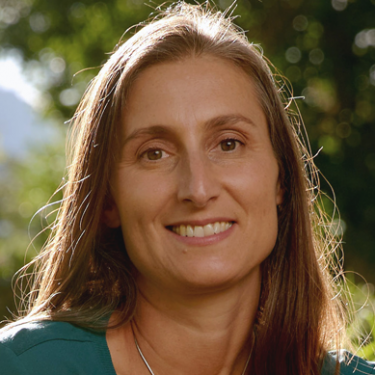
Dr. Claire Monteleoni (University of Colorado, Boulder)
Deep Unsupervised Learning for Climate Informatics
Abstract: Despite the scientific consensus on climate change, drastic uncertainties remain. Crucial questions about regional climate trends, changes in extreme events, such as heat waves and mega-storms, and understanding how climate varied in the distant past, must be answered in order to improve predictions, assess impacts and vulnerability, and inform mitigation and sustainable adaptation strategies. Machine learning can help answer such questions and shed light on climate change. I will give an overview of our climate informatics research, focusing on challenges in learning from spatiotemporal data, along with semi- and unsupervised deep learning approaches to studying rare and extreme events, and precipitation and temperature downscaling.
Bio: Claire Monteleoni is an Associate Professor, and the Associate Chair for Inclusive Excellence, in the Department of Computer Science at the University of Colorado Boulder, and the founding Editor in Chief of Environmental Data Science, a Cambridge University Press journal, launched in December 2020. She joined CU Boulder in 2018, following positions at University of Paris-Saclay, CNRS, George Washington University, and Columbia University. She completed her PhD and Masters in Computer Science at MIT and was a postdoc at UC San Diego. She holds a Bachelor's in Earth and Planetary Sciences from Harvard. Her research on machine learning for the study of climate change helped launch the interdisciplinary field of Climate Informatics. In 2011, she co-founded the International Conference on Climate Informatics, which turned 10 years old in 2020, and has attracted climate scientists and data scientists from over 20 countries and 30 U.S. states. She gave an invited tutorial: Climate Change: Challenges for Machine Learning, at NeurIPS 2014.
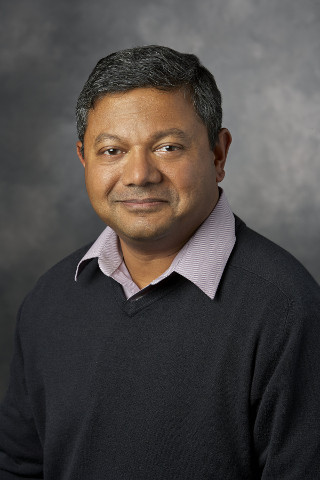
Dr. Arun Majumdar (Stanford University)
Energy, Climate and Sustainability: The Defining Issue of the 21st Century
Abstract: The 20th Century was marked by dramatic innovations in medical care, transportation, food production, communications, computing, aerospace technology and the built environment. This has led to remarkable improvements in our quality of life, brought billions of people out of poverty, and has supported a four-fold increase in global population and seven-fold increase in GDP per capita. The fundamental infrastructure to enable these historic changes was energy. The use of fossil fuels and the associated greenhouse gas emissions has made this 20th century approach unsustainable. We are now witnessing a global economy undergo a once-in-a-century shift to chart a sustainable course. Will this happen sufficiently fast to address climate change? What are the innovations needed? How can computing enable and empower this transition? Will it happen uniformly around the world? What are the roles of academic institutions, government, business, and non-profits? This talk will explore these issues in depth and breadth and take stock of the opportunities and challenges that lie ahead of us.
Bio: Dr. Arun Majumdar is the Jay Precourt Provostial Chair Professor at Stanford University, a faculty member of the Department of Mechanical Engineering.
From 2009 to 2012, Dr. Majumdar served as the Founding Director of ARPA-E and from March 2011 to June 2012 as the Acting Under Secretary of Energy. After leaving Washington, Dr. Majumdar was the VP for Energy at Google.
Dr. Majumdar is a member of the National Academy of Sciences, National Academy of Engineering and the American Academy of Arts and Sciences. He also served as the Vice Chairman of the Advisory Board to the US Secretary of Energy, Dr. Ernest Moniz, was a Science Envoy for the US Department of State. He currently serves as the Chair of Advisory Board of the US Secretary of Energy, Jennifer Granholm, as well as on the advisory board of numerous energy businesses and non-profits.
Dr. Majumdar received his BS in Mechanical Engineering in 1985 from the Indian Institute of Technology, Bombay, and his Ph.D. from the University of California, Berkeley in 1989.
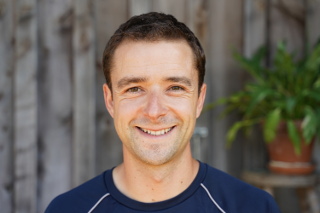
Dr. Victor Anton (Founder and CEO of Wildlife.ai)
Wildlife.ai — Using artificial intelligence to accelerate wildlife conservation
Abstract: While Artificial Intelligence (AI) is revolutionising multiple aspects of our lives, there is limited uptake of AI in supporting ecological well-being and protecting the nearly 1 million species currently threatened with extinction. Wildlife.ai is a New Zealand not-for-profit aiming to bridge this gap between computer sciences and wildlife conservation worldwide. This presentation will provide an overview of how Wildlife.ai combines machine learning, community science, and environmental education to provide accurate and timely information to biologists and conservation managers. From developing open-source machine learning models and data analysis apps to creating educational activities about AI and wildlife conservation. Wildlife.ai works to democratise the use of AI and enhance current conservation efforts.
Bio: Victor has extensive experience in conservation biology, wildlife monitoring and ecological modeling. He discovered the strengths and limitations of machine learning for conservation while working with camera traps during his PhD at Victoria University of Wellington, New Zealand. Since then, he has worked with numerous research institutions, governments and communities around the world to translate big data into scientific discoveries and better conservation management practices.
- Chairs
- Ayan Mukhopadhyay (Vanderbilt University)
- Chris Yeh (California Institute of Technology)
- Katelyn Morrison (Carnegie Mellon University)
- Steering Committee
- Douglas Fisher (Vanderbilt University)
Thanks to CompSustNet, the Cornell Institute for Computational Sustainability (ICS), and the National Science Foundation for sponsoring CompSust-2022.